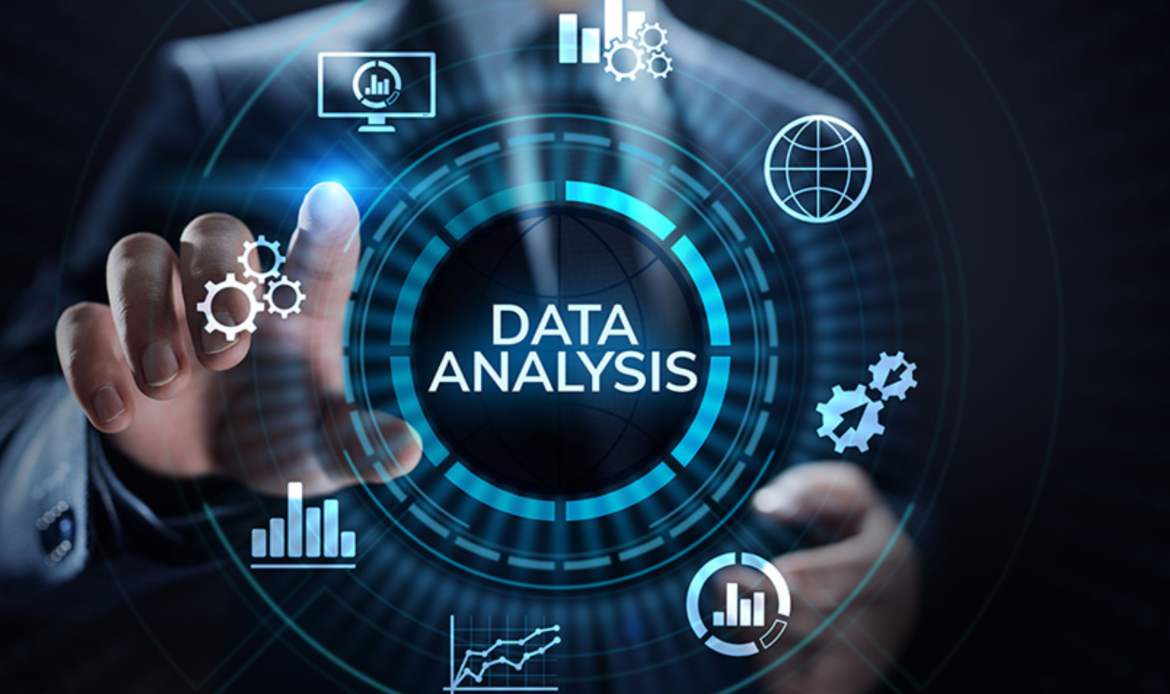
Data Analytics
Data analytics is a transformative process that turns raw data into meaningful insights, enabling informed decision-making and driving strategic initiatives. It encompasses a range of techniques and methodologies to process and analyze data, offering valuable perspectives across various domains. Below is a comprehensive overview of data analytics, its core components, techniques, tools, and applications.
1. Core Components of Data Analytics
a. Data Collection
The initial step in data analytics involves gathering data from multiple sources. This data can be:
- Internal Sources: Customer databases, financial records, operational logs.
- External Sources: Social media, public datasets, market research.
b. Data Storage
Collected data needs to be stored in a manner that allows for efficient retrieval and analysis.
- Databases: Structured data storage like SQL databases (e.g., MySQL, PostgreSQL).
- Data Warehouses: Centralized repositories designed for query and analysis (e.g., Amazon Redshift, Google BigQuery).
- Data Lakes: Storage systems that handle large volumes of raw, unstructured data (e.g., Hadoop, Azure Data Lake).
c. Data Processing
Transforming raw data into a format suitable for analysis through cleaning, integrating, and organizing.
- ETL Processes: Extract, Transform, Load operations to prepare data (e.g., Talend, Apache Nifi).
- Data Wrangling: Techniques to clean and prepare data for analysis (e.g., Python Pandas, R dplyr).
d. Data Analysis
Applying statistical and computational techniques to uncover patterns and insights.
- Exploratory Data Analysis (EDA): Initial data examination to summarize characteristics and uncover patterns (e.g., visualizations, summary statistics).
- Advanced Analytics: Techniques like predictive modeling, machine learning, and data mining.
e. Data Visualization
Representing data through graphical formats to simplify understanding and communication.
- Dashboards: Interactive interfaces displaying key metrics and trends (e.g., Tableau, Power BI).
- Graphs and Charts: Visual elements like bar charts, line graphs, and scatter plots to represent data relationships.
f. Data Interpretation
Drawing actionable conclusions from the analyzed data to support decision-making.
- Reporting: Presenting findings in a structured manner for stakeholders.
- Decision Support: Using insights to guide business strategies and actions.
2. Types of Data Analytics
a. Descriptive Analytics
Summarizes historical data to understand what has happened.
- Methods: Aggregation, trend analysis, and data summarization.
- Examples: Sales performance reports, user activity logs.
b. Diagnostic Analytics
Investigates the reasons behind past outcomes.
- Methods: Drill-down analysis, root cause analysis, and correlation.
- Examples: Analyzing factors contributing to a sales decline, customer churn analysis.
c. Predictive Analytics
Forecasts future outcomes based on historical data.
- Methods: Machine learning algorithms, time series analysis, regression models.
- Examples: Demand forecasting, risk assessment models.
d. Prescriptive Analytics
Recommends actions to achieve desired results or optimize outcomes.
- Methods: Optimization techniques, simulation models, decision analysis.
- Examples: Supply chain optimization, personalized marketing strategies.
e. Exploratory Data Analysis (EDA)
Examines data sets to uncover underlying patterns without predefined hypotheses.
- Methods: Data visualization, clustering, and dimensionality reduction.
- Examples: Identifying new customer segments, exploring potential correlations in a dataset.
3. Data Analytics Techniques
a. Statistical Analysis
Applying statistical methods to summarize and infer properties of data.
- Descriptive Statistics: Measures like mean, median, and standard deviation to summarize data.
- Inferential Statistics: Techniques like hypothesis testing and regression analysis to draw conclusions from sample data.
b. Data Mining
Extracting useful information and patterns from large datasets.
- Association Rules: Discovering relationships between variables in large datasets.
- Clustering: Grouping data points with similar characteristics.
- Classification: Assigning data points to predefined categories based on attributes.
c. Machine Learning
Creating models that can learn from data and make predictions or decisions.
- Supervised Learning: Training models on labeled data to make predictions (e.g., linear regression, decision trees).
- Unsupervised Learning: Identifying patterns without labeled outcomes (e.g., K-means clustering, principal component analysis).
- Reinforcement Learning: Learning to make decisions through rewards and penalties over time.
d. Data Visualization
Converting data into visual formats to make insights accessible and understandable.
- Charts and Graphs: Bar charts, line graphs, scatter plots, and heatmaps.
- Dashboards: Consolidating various visual elements into a single interface for real-time data monitoring.
- Geospatial Visualization: Mapping data to geographical locations for spatial analysis.
4. Tools and Technologies in Data Analytics
a. Data Analysis Tools
- Excel: Widely used for basic data manipulation and analysis.
- SQL: Essential for querying and managing relational databases.
- Python: Powerful for data analysis and machine learning (libraries like Pandas, NumPy, scikit-learn).
- R: A statistical programming language popular in academia and research.
b. Business Intelligence (BI) Tools
- Tableau: Data visualization tool known for creating interactive and shareable dashboards.
- Power BI: Microsoft’s tool for data visualization and business analytics.
- QlikView: Platform for developing dynamic and interactive visualizations.
c. Data Processing Frameworks
- Apache Hadoop: Framework for distributed storage and processing of large datasets.
- Apache Spark: Engine for big data processing with built-in modules for streaming, SQL, and machine learning.
- ETL Tools: Tools like Talend, Informatica, and Apache Nifi for extracting, transforming, and loading data.
d. Machine Learning Platforms
- TensorFlow: Open-source framework for building machine learning models.
- scikit-learn: Python library for data mining and machine learning.
- Azure Machine Learning: Microsoft’s cloud-based service for building and deploying machine learning models.
e. Cloud-Based Data Solutions
- Amazon Web Services (AWS): Comprehensive cloud platform offering services for data storage, processing, and analysis.
- Google Cloud Platform (GCP): Cloud services including BigQuery for data warehousing and AI tools.
- Microsoft Azure: Cloud platform providing a range of services for data analytics and AI.
5. Data Governance and Ethics
a. Data Governance
Establishing policies and practices to manage data effectively and securely.
- Data Quality: Ensuring accuracy, consistency, and reliability of data.
- Data Security: Protecting data from unauthorized access and breaches.
- Data Privacy: Adhering to regulations like GDPR and CCPA to protect personal information.
b. Ethics in Data Analytics
Ensuring responsible use of data to avoid bias, discrimination, and privacy violations.
- Bias and Fairness: Preventing algorithms from perpetuating or amplifying biases.
- Transparency: Being clear about data collection, usage, and interpretation.
- Accountability: Taking responsibility for the outcomes of data-driven decisions.
6. Applications of Data Analytics
a. Business and Marketing
- Customer Insights: Analyzing customer behavior to enhance targeting and personalization.
- Sales Optimization: Forecasting demand and optimizing pricing strategies.
- Supply Chain Management: Improving operational efficiency and inventory management.
b. Healthcare
- Patient Care: Using data to improve diagnosis and treatment plans.
- Operational Efficiency: Streamlining hospital operations and resource allocation.
- Disease Prediction: Applying predictive analytics to identify potential health risks.
c. Finance
- Fraud Detection: Identifying and preventing fraudulent transactions.
- Risk Management: Assessing and mitigating financial risks.
- Portfolio Optimization: Analyzing market data to maximize investment returns.
d. Retail
- Personalized Recommendations: Suggesting products based on customer preferences and behavior.
- Inventory Management: Predicting demand to maintain optimal stock levels.
- Customer Experience: Improving service and engagement through data analysis.
e. Public Sector
- Policy Making: Using data to inform and evaluate public policies.
- Public Safety: Analyzing crime data to allocate resources and prevent incidents.
- Urban Planning: Utilizing data to optimize city infrastructure and services.
7. Future Trends in Data Analytics
a. AI and Machine Learning Integration
- Automated Analytics: Using AI to automate data analysis and generate insights.
- Augmented Analytics: Enhancing human analysis with AI-driven insights and recommendations.
b. Real-Time Analytics
- Streaming Data: Analyzing data as it is generated for immediate insights.
- Edge Analytics: Processing data at the edge of the network to reduce latency and bandwidth usage.
c. Big Data and Scalability
- Scalable Solutions: Developing tools and platforms capable of handling the growing volume, velocity, and variety of data.
- Hybrid Architectures: Combining on-premise and cloud solutions for effective data management and analysis.
d. Enhanced Data Privacy and Security
- Privacy-Preserving Techniques: Implementing methods like differential privacy to analyze data while protecting individual privacy.
- **Blockchain for